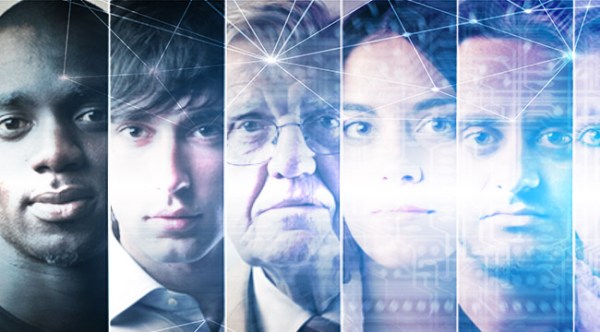
The idea of a computer being racist ought to sound absurd. A computer is a hunk of silicon that doesn’t form opinions (bigoted or otherwise). But this notion ignores a crucial flaw in reasoning: Computers only understand the world through the data we give them. They are hunks of silicon, programmed by us, and we are inherently flawed. Which helps explain why, increasingly, the data is turning up ugly truths about human nature that, if we’re not careful, might affect how we do everything from identify photos to decide criminal sentencing.
How Computers Become Racist
In 2010, Joz Wang bought a Nikon point and shoot camera for her parents. While messing around with it, she noticed something odd: The camera’s face recognition software seemed to be malfunctioning, asking if someone in the photo had blinked every time she took a picture. It wasn’t until her brother made a “bug-eyed” face and the warning vanished that she realized the camera literally didn’t understand her facial structure.
The tech industry loves algorithms and big data, but that data is generated entirely by human beings, and that means human fallacies and even outright hatred can surface quite easily. Until it was spotted and filtered out by Google, punching the world’s most inflammatory racist term into Google Maps would give you directions to the White House. HP also got in hot water because their face-tracking software was unable to see black people — which inspired an entire episode of the sitcom Better Off Ted.
These incidents proved to be warnings, which ought not to have been ignored, because algorithms are designed to observe, and they can just as easily learn the worst from us as the best. Microsoft figured this out the hard way with Tay, a chatbot it debuted on Twitter that was promptly taught by trolls to spew racist vitriol. Learning algorithms are great, right up until they learn terrible behavior from us.
Racist Computers And Criminal Justice
Increasingly, the justice system is turning to past data to figure out which criminals are a danger and which are more likely to be rehabilitated. If that data were unbiased and clear, that would be one thing. Unfortunately, as anyone with even a passing awareness of race in America can tell you, the justice system has a long history of racism and its use of algorithms reflects that ugly past.
ProPublica recently audited the results of an algorithm used by Broward County, Florida, to see how effective it was at predicting future misbehavior. The overall results were unimpressive: The algorithm was only accurate roughly 20% of the time at predicting violent second offenses, and 61% accurate at predicting recidivism in general. But two very striking trends emerged from the data: White offenders were consistently ranked as a much lower risk than black offenders, who got “false flags” at roughly twice the rate of whites.
It’s worth noting that ProPublica doesn’t think this algorithm was coded with any sort of malicious intent. In fact, the algorithm deliberately omits any consideration of race in an attempt to avoid just these results. But, again, all the algorithm has to base its decisions on is the data it’s been fed.
Simply put, unless humans notice flaws in the data, those flaws will slip through and be reflected in the algorithm. For example, it’s technically accurate to say that non-white people are arrested at four times the rate of white people for marijuana possession. To an algorithm, that data says “Non-white people are more likely to be drug offenders.” But there’s another explanation: White people tend to be treated differently, often preferentially by the police, even when crimes occur among both at the same rates. But if the algorithm doesn’t have that data (which is based on individual interactions and therefore virtually unquantifiable), it has no way of coming to a conclusion that to is patently obvious to a human.
A poor data diet means poor results, and poor results, in this case, might lead to disproportionate sentences to non-violent offenders. Worse, there’s no way for defendants to evaluate or challenge this score: The algorithm is proprietary and what it considers and how it weighs its data are closely guarded corporate secrets.
A Reminder That Data Is Not Intelligence
Algorithms aren’t good or bad. Like any technology, they only go wrong when we misuse them, accidentally or with intent. What we’re seeing now is a clear sign that our data is flawed and, thus, that our algorithms are flawed. And to be fair, it can be difficult to accept. If our data is wrong, after all, that means the tools we used to gather it are wrong, that our working assumptions need to be carefully addressed and reevaluated. It’s a big project and a deeply scary thought for many people.
But clearly, as we move further into a tech-centric future, removing racial bias from our data and algorithms is of utmost importance. Because if we don’t correct the problems our algorithms are accidentally revealing to us, we could potentially cause a staggering amount of misery.